Reducing Alpha Decay with AI Predictive Signals
The electronic trading industry moves quickly from innovation to innovation. Yesterday’s cutting-edge technology becomes tomorrow’s table stakes all too soon.
Trading signals are the latest engine in the drive for faster, more responsive trading. As the machine learning technology that underpins it gains popularity, firms risk “alpha decay,” also known as “alpha bleed,” as a result of more crowded trades. While AI-generated predictive signals—both in-house and vendor-provided—are a valuable way to enhance alpha, firms should be aware of the potential for crowding issues and develop strategies to mitigate them.
Lost in a crowd: The risk of alpha decay
Crowded trades are nothing new. It’s possible to have many participants crowding onto a trade based solely on trend-following or passive investment strategies. However, the automated nature of algorithmic trading poses special challenges.
Separate algorithms created by different firms are unlikely to result in identical predictive signals, although they could easily track similar trends. If a number of firms use the same signal service, the possible risk of trying to make the same trades is higher. The potential alpha indicated by a signal could erode as others attempt to enter the trade. Whether you call it alpha bleed or alpha decay, firms must consider the potential impact.
Alpha capture can be seen by some as a zero-sum game; the first to the line takes the prize. In fact, a lot depends on the type of alpha generated. Most firms seek robust alpha that can stand up under variable conditions, even as independent market participants begin to simultaneously trade the same signal.
So, avoiding predictive signals in order to prevent alpha decay isn’t the answer—that just cedes the field to those who continue to use them. What is required are strategies that leverage signals’ utility while enhancing and monitoring their impact.
The journey from speed to prediction
We’ve been here before.
Beginning in the early 2000s, speed became an important differentiator for firms who kept investing in new technology to improve the latency of market data delivery and trading. From direct feeds to co-location to FPGAs and wireless wide area networking (WAN), the goal was to shave latencies to microsecond-, and then sub-microsecond speeds for the fastest market participants—high-frequency traders (HFTs).
Once a critical mass of HFTs had achieved these speeds, their speed-based alpha advantage began to erode. There was obviously no turning back to the days before the “race to zero,” but firms began to search for some way to build on low-latency trading to gain the next advantage.
Enterprising firms began using machine learning to study patterns of market data in real time, using them to predict imminent price and liquidity changes. While it originally was limited to large institutional investors with the resources to hire teams of data scientists, signal services have begun to democratize that technology, making it available more widely.
The change has already been profound: A 2020 survey of 107 global capital markets professionals showed that 44% used artificial intelligence (including machine learning); another 17% expected to be using it 12-24 months out.
How to keep all those new adopters from eating into your alpha? The answer requires a measurable and flexible approach. Firms need the ability to quantify the performance of predictive signals daily, if not in real time. They also need the ability to set and change parameters on signals to optimize those that are most useful to their strategies. Finally, firms must scale and accelerate their adoption of signals by integrating signal services. Those that do can invest more effort in their own algorithms and proprietary signals, using signal services as a foundation for more sophisticated strategies to stay ahead of the pack.
Some alpha decay from signals is inevitable. But like latency before it, signals will become foundational sources of data that allow firms to refine existing strategies while staying in the hunt for the next trading frontier.
Setting parameters for signals
One of the most important decisions to make in using signals is setting parameters for when to act. As an example, Exegy’s Signum suite of signals includes Quote Vector, which predicts the direction of the next price change in the NBBO for US-listed equities. This results in over two billion signals a day delivered as pairs of probabilities: The probability that the next change to the best bid will be up, and the probability that the next change to the best offer will be up. The probability of downward price movements are simply the complement of those values.
Firms tailor their use of Quote Vector by choosing thresholds at which the signal is “fired”—when a directional prediction is made because the probability exceeded the threshold. A strategy that seeks out fewer, more likely outcomes would choose a higher threshold, while one that looks for greater frequency would choose a lower one.
This allows firms to manage risk for different trading strategies by tuning signal accuracy. In addition, firms using the same signal service can set thresholds that differentiate their signals, helping to prevent crowding.
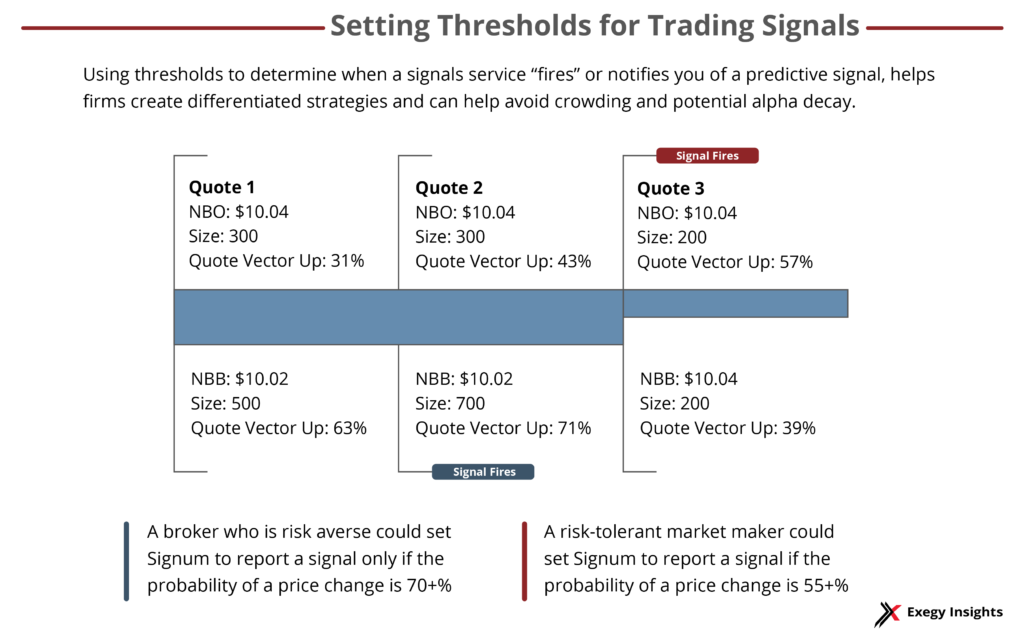
A signal/algorithm mix
Firms with in-house data scientists can build on the use of signal services by employing them in tandem with their own algorithms to seek out new, unmined alpha.
An example where predictive signals can add value is the volume-weighted average price or VWAP execution algorithm. A VWAP algo can help spread out a large order to minimize its effect on the market, by targeting an execution price pegged to the VWAP price curve. Signals that predict the duration and direction of price changes (such as Signum’s Quote Fuse and Quote Vector) can help inform a VWAP algorithm. Improving the ability to predict quote changes in real-time aids in reducing trading slippage, which maximizes alpha and increases its robustness. Additional proprietary signals can be used to further optimize performance, for example by predicting the volume profile in the next time interval.
An exchange or alternative trading system (ATS) can use signal services to create proprietary order types that leverage the signals’ ability to predict price movements. For example, a trading venue could offer an order which is displayed on the book at one price while using signals to seek liquidity within a more aggressive price range. Like other types of non-displayed orders, it offers the opportunity for additional liquidity and price improvement after visible orders at a price level have been exhausted.
A signal (such as Quote Vector) that predicts the direction of the next NBBO price movements could help manage the non-displayed discretionary range (or offset) to optimize execution.
How robust is your alpha? Monitoring for decay
Regardless of the approach, minimizing alpha decay requires constant monitoring: You can’t manage what you don’t measure.
Studies have shown that alpha on new trades decays in about 12 months on average. Developing robust alpha will allow first movers to keep reaping profits while others adopt similar signals. One measure of an alpha’s robustness is its performance under “crowded” conditions with increased trading volume. Alpha that quickly fades away as volume spikes is less robust than alpha that doesn’t show material degradation in any of its benchmarks.
Among the most common benchmarks for measuring the robustness of alpha are:
- Returns and the standard deviation of returns.
- Sharpe ratio, which is the return on investment compared to a risk-free return.
- Information ratio, the risk-adjusted return relative to a benchmark such as the S&P 500.
- Max drawdown, or the maximum observed loss from a peak to a low during a specific period.
Monitoring movement on these benchmarks will help firms decide whether to abandon a signal or change it in some way.
A state-of-the-art signal service provides the transparency and tools to achieve robust alpha. It must continuously monitor its signals to ensure that they retain expected performance under changing market conditions. Traders must also be provided with guidance and the ability to modify their strategies’ usage of the signals to continuously optimize performance. Exegy’s Signum service ticks all these boxes.
Want more information about Signum? Explore our whitepapers, or request demo data. For a more detailed discussion of how to use Signum signals in concert with other algorithms to capture and preserve alpha, contact us.
Want to learn more about Signum real-time signals? Learn how they can infuse a standard VWAP algo with predictive intelligence.